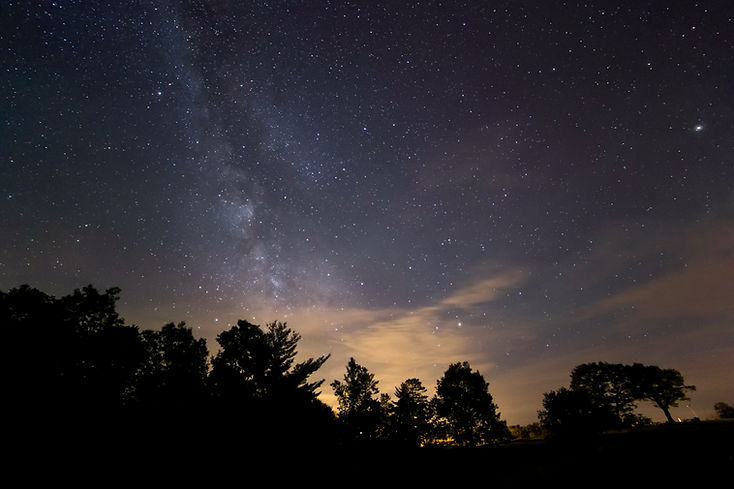
Pravish Sainath
PhD Student in Machine Learning
Université de Montréal
Montréal, Canada
About Me
I'm a PhD student at Université de Montréal.
​
I am advised by Prof. Guillaume Lajoie.
The purpose of my life and study is to better understand the nature and mechanisms of both natural and artificial intelligence.
I am mostly interested in using computational and cognitive neuroscience to improve deep and reinforcement learning methods and applying deep learning methods to better understand the brain.
Before this, I was an engineer at the startup Hammerhead, working on computing cycling fitness metrics from smart cycling sensors for their flagship product 'Karoo' and developing navigation solutions for the platform.
I obtained my masters degree in Computer Science from Université de Montréal and undergraduate degree in Computer Science and Engineering from Anna University in Chennai, India.
Full CV
Research Interests
I am broadly interested in the intersection of the two disciplines that fascinate me: artificial intelligence and neuroscience.
My tentative thesis project is to use neuroimaging data corresponding to a visual identification task to build and analyze generative models of artificial neural networks that are capable of producing the same response as the human brain for the visual stimuli.
I am broadly interested in the intersection of the two disciplines that fascinate me: artificial intelligence and neuroscience.
My tentative thesis project is to use neuroimaging data corresponding to a visual identification task to build and analyze generative models of artificial neural networks that are capable of producing the same response as the human brain for the visual stimuli.
My general research directions are :
​
Deep Learning for neuroimaging
Neuroinformatics
Neuroscience inspired AI
Computational Neuroscience
Cognitive Robotics
I have previous experience working in a research project in using machine learning for interpreting fMRI images
Projects
Faster music generation in SampleRNN using Inverse Autoregressive Flow (IAF)
Studied flow-based generative models.
Used inverse autoregressive flow transform to generate music using a SampleRNN architecture
Demonstrated a better likelihood for this method
Paper
Visual Odometry : Classical methods, Deep methods and beyond
Visual odometry is the process of estimating the motion of a moving agent using only the visual information captured.
This was a course project for the Duckietown course in which reviewed different approaches to this VO problem and implemented in the Duckietown environment.
More about the VO experiments we ran
Notes about the fundamental concepts in Visual Odometry that I contributed for the Duckiebook
Investigation of depth and residual layers in deep neural networks
Studied the effect of residual layers in deep neural networks